AI in Cyber Espionage
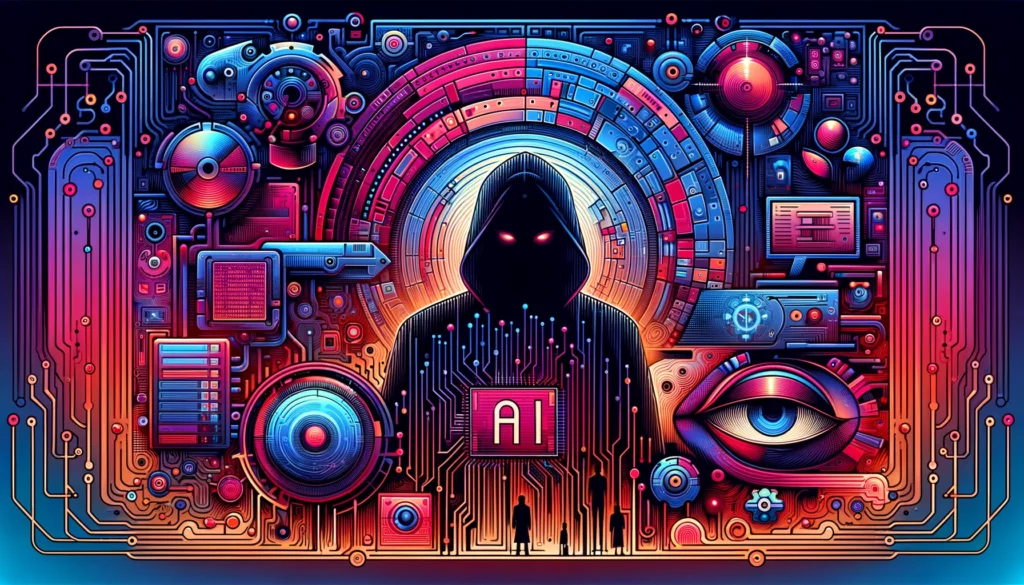
The integration of Artificial Intelligence (AI) in the sphere of cyber espionage and counterintelligence has began new stage of technological sophistication and complexity. AI technologies have become powerful tools used by both enemies and defenders, reshaping the field of digital espionage and security measures.
AI increased the capabilities of state-sponsored cyber espionage operations. Adversarial nations use AI-powered algorithms to conduct accurately planned and stealthy attacks on critical infrastructure, government agencies, corporations, and individuals. These advancements enable opponents to conduct sophisticated reconnaissance, automate target identification, and execute deceptive tactics, making cyber espionage operations more indefinite and challenging to detect. In response to the escalating threats posed by AI-driven cyber espionage, defenders employ AI technologies for cybersecurity counterintelligence.
The symbiotic relationship between AI and cyber espionage signifies a continuous technological arms race, where adversaries constantly refine their methods using AI-driven innovations, while defenders harness AI to strengthen cyber defenses and reduce increasing threats. However, ethical considerations, responsible usage, and collaborative efforts among security experts remain crucial in harnessing the potential of AI to safeguard digital infrastructures against increasingly sophisticated cyber threats.
Evolution of Cyber Warfare
Today state-to-state aggression isn’t confined to traditional military, economic, or political means alone. Cyber operations have emerged as a potent tool for hostile actions between nations, posing an ever-growing threat in an expansive and relentless digital battleground. This form of technological warfare, known as cyber warfare, endangers national security by exploiting technology to breach critical systems and steal valuable intelligence.
Cyber warfare encompasses various tactics, including hacking or disrupting a nation’s vital infrastructure and infiltrating intelligence repositories for strategic data. With technology’s evolution, cyber warfare has escalated to become an existential threat to national security, especially as technological advancements continue to fuel the frequency and complexity of cyber-attacks.
Artificial Intelligence plays crucial role in shaping the cyber warfare landscape. These advancements augment the cyber domain’s capabilities, offering significant advantages but also harboring the potential to intensify cyber threats to unprecedented levels in the future.
Cyber warfare, orchestrated by governments, aims to wreak havoc on computer systems, conduct espionage, or disrupt adversaries’ critical infrastructure. The increasing reliance on technology has amplified the frequency and severity of cyber warfare attacks. The Morris Worm, one of the earliest documented cyberattacks, affected approximately six thousand computers, resulting in damages ranging from hundreds of thousands to millions of dollars. Targeting specific Unix Operating System versions, this worm significantly disrupted computer functions and communications, causing widespread destruction.
Another notable instance is the Stuxnet Worm, deployed against the Iran Nuclear Program. Designed to target Windows systems, Stuxnet destroyed nearly a thousand uranium enrichment centrifuges, effectively stalling Iran’s nuclear weapons development. Rapidly spreading through networks, Stuxnet compromised critical information, impacting the Iranian facility’s operations. Encapsulated within a USB drive, the worm initiated its destructive sequence upon insertion into a computer at the nuclear weapons facility.
These examples underscore the potency of cyber warfare and the devastating impact it can have on critical systems, emphasizing the urgency for heightened cybersecurity measures in an increasingly digitized world. But for fortifying security measures it is needed to know what kind of instruments are used for attacks.
Sophisticated Attacks
State-sponsored cyber espionage operations have evolved with the integration of AI. Adversarial nations use AI-driven techniques to conduct stealthy, targeted attacks on critical infrastructure, government agencies, corporations, and even individuals.
AI-Powered Stealth and Targeting. AI algorithms are used for conducting stealthy and precise attacks on critical targets. AI enables to camouflage activities within normal network traffic, making such operations harder to detect. This stealthiness allows to navigate through defenses more effectively.
Automated Reconnaissance and Vulnerability Identification. AI-driven tools aid in automated reconnaissance by sifting through vast amounts of data to identify potential vulnerabilities in systems and networks. Machine learning algorithms analyze network structures, system configurations, and historical data to pinpoint weaknesses that can be exploit for infiltration or data exfiltration.
Adaptive and Targeted Attacks. AI algorithms enable to adapt their strategies dynamically. They learn from ongoing interactions with a target system, allowing for the customization of attack vectors to bypass specific security measures.
AI to tailor their attacks to the unique characteristics of each target, increasing the precision and efficacy of their infiltration attempts.
Enhanced Social Engineering and Phishing. AI-powered social engineering techniques aid in creating highly convincing phishing attacks and deceptive campaigns. Natural Language Processing (NLP) models generate convincing, personalized messages that trick individuals into revealing sensitive information or granting unauthorized access to systems.
Machine Learning for Weaponized Malware. Usage of machine learning algorithms to design and refine malware capable of evading traditional signature-based detection systems. These AI-generated or AI-enhanced malware variants are engineered to mutate and evolve, making them more challenging to detect and mitigate.
AI-Driven Evasion of Defense Mechanisms. Attackers employ AI to analyze and evade security controls such as firewalls, intrusion detection systems (IDS), and antivirus software. AI models iteratively refine attack strategies, identifying vulnerabilities in defensive measures and circumventing them by exploiting their blind spots.
The integration of AI in state-sponsored cyber espionage operations amplifies capabilities to conduct intricate, targeted, and stealthy attacks on high-value targets, posing significant challenges to traditional cybersecurity defenses. AI-driven techniques enable to evolve their attack methodologies, adapt to defenses, and execute more sophisticated infiltration strategies.
Enhanced Reconnaissance
AI-powered algorithms make easier extensive data gathering and analysis. These tools enable to conduct precise reconnaissance, identify vulnerabilities, and tailor attacks for maximum impact. AI-powered algorithms play a crucial role in boosting the reconnaissance phase of cyber espionage operations, providing adversaries with sophisticated capabilities for data gathering, analysis, and attack tailoring.
Automated Data Collection. AI-driven algorithms scour various data sources, including open-source intelligence (OSINT), social media, databases, and web platforms, to collect huge amounts of information. Natural Language Processing (NLP) models parse and extract relevant data from unstructured sources like news articles, social media posts, forums, and websites, aiding in the collection of valuable intelligence.
Data Analysis and Pattern Recognition. Machine learning algorithms process and analyze the collected data to identify patterns, trends, and potential vulnerabilities across disparate sources. AI-powered analytics tools can discern correlations between seemingly unrelated data points, revealing critical insights about potential weaknesses or exploitable gaps in target systems or networks.
Predictive Analytics for Vulnerability Identification. AI models use historical data and behavior patterns to predict potential vulnerabilities or weaknesses in target systems. Predictive analytics algorithms forecast possible attack vectors, enabling adversaries to anticipate and exploit vulnerabilities before they are detected and patched by defenders.
AI-Enhanced Target Profiling. Machine learning techniques aid in creating detailed profiles of target entities, including their infrastructure, software configurations, user behaviors, and network architecture. AI algorithms generate comprehensive maps of interconnected systems, facilitating the identification of high-value assets and potential entry points for infiltration.
Tailoring Attack Strategies. AI-powered analytics provide insights to tailor attack strategies based on the specific vulnerabilities and weaknesses identified during reconnaissance. The intelligence is used to craft highly targeted and exact attack vectors, optimizing the chances of successful infiltration or data exfiltration.
Automated Threat Intelligence Gathering. AI-driven threat intelligence platforms continuously collect and analyze threat data, enriching understanding of developing security field and potential attack vectors. These platforms use machine learning to process threat feeds, security reports, and incident data, enabling to stay updated on emerging vulnerabilities and exploit them effectively.
AI-powered reconnaissance empowers adversaries to conduct comprehensive and precise data collection, analysis, and prediction, providing them with critical intelligence to craft tailored attack strategies for maximum impact. This level of sophistication in reconnaissance significantly heightens the effectiveness and stealth of cyber espionage operations.
Automated Targeting
AI algorithms aid in the automated identification of high-value targets, allowing to streamline their efforts and focus on critical assets, intellectual property, or sensitive information. The use of AI algorithms in automated targeting plays a key role in enabling to efficiently identify and prioritize high-value targets within cyber espionage operations.
AI-Powered Target Prioritization. Machine learning algorithms analyze vast datasets to identify potential high-value targets based on various parameters such as the value of assets, criticality of systems, or strategic significance. AI models assess indicators like system configurations, user behaviors, network traffic, or data sensitivity to rank targets according to their potential impact on achieving attackers’ objectives.
Predictive Analysis for Target Selection. AI-driven predictive analytics utilize historical data and patterns to forecast the potential impact and value of targeting specific entities. These algorithms predict the likelihood of successful infiltration, data exfiltration, or the strategic importance of compromising particular targets.
Contextual Analysis and Decision Support. Natural Language Processing (NLP) models analyze textual data from various sources to gather context about potential targets, such as corporate reports, employee communications, or public statements. AI algorithms interpret and contextualize information, providing decision support to attackers by identifying targets aligned with their objectives.
Behavioral Analysis for Targeting. Machine learning techniques perform behavioral analysis on user actions, system interactions, or network behaviors to identify targets that exhibit characteristics of vulnerability or exploitation potential.
AI models recognize anomalies or deviations from normal behaviors within target entities, flagging them as potential high-value targets for further exploitation.
Adaptive Targeting Strategies. AI-driven algorithms continuously adapt target selection strategies based on real-time feedback and developing intelligence gathered during reconnaissance and analysis phases. Usage of machine learning to refine targeting approaches, dynamically adjusting focus areas based on the success or failure of previous targeting efforts.
Resource Optimization through AI Targeting. Automated targeting enables to allocate resources more efficiently by focusing efforts on high-priority targets with maximum strategic or exploitative value.
AI-enhanced targeting streamlines efforts, optimizing resource allocation for increased effectiveness in achieving their espionage objectives. AI-powered automated targeting facilitates the intelligent selection and prioritization of high-value targets within cyber espionage operations.
Deceptive Tactics
AI-driven deception techniques, such as generating sophisticated phishing attacks or creating realistic fake personas, augment cyber espionage efforts, making them more convincing and challenging to detect. The integration of AI in cyber espionage introduces advanced tactics, using AI-driven techniques to enhance the sophistication and stealth of attacks.
AI-Generated Phishing Attacks. Natural Language Processing (NLP) models enable the creation of highly convincing and personalized phishing emails, messages, or content. AI algorithms analyze and replicate writing styles, linguistic patterns, and communication behaviors to craft phishing messages that mimic genuine communications, increasing their effectiveness in deceiving targets.
Spear Phishing with AI. Machine learning algorithms process vast amounts of data about potential targets, including social media profiles, employment history, and personal information. AI-powered systems generate targeted spear phishing campaigns that use detailed knowledge about individuals, making the messages highly personalized and tailored to the recipient’s interests or roles within an organization.
Deepfake Technology for Impersonation. AI-driven deepfake technology fabricates realistic audio, video, or text content, enabling to impersonate individuals or manipulate media to spread misinformation. Generative models use neural networks to create convincingly altered media content, imitating real voices, faces, or writing styles, which are emploied for impersonation or disinformation purposes.
AI-Powered Social Engineering. Adversaries utilize AI algorithms to analyze and predict human behavior, personality traits, and decision-making patterns based on available data. Machine learning models simulate human-like interactions, allowing to engage with targets in conversations that appear genuine, fostering trust and increasing the success rate of social engineering attacks.
Automation of Fake Persona Creation. AI systems automate the creation of fake online personas, including social media profiles, biographies, and interaction histories. Machine learning is used to generate entire digital identities, complete with background stories, connections, and activities, establishing a false sense of legitimacy and trustworthiness.
Adaptive Deception Techniques. AI-enhanced deception tactics continually develop by learning from successful or failed attempts.
Adversaries iteratively refine deceptive strategies based on feedback and analytics, adapting to security measures or changes in target behavior to avoid detection.
The usage of AI-driven deception techniques in cyber espionage empowers to create highly convincing and personalized deceptive content, including phishing attacks, impersonations, and fabricated personas. These advanced tactics increase the sophistication and challenge of detecting malicious activities, amplifying the effectiveness of cyber espionage efforts.