AI-Powered Threat Hunting and Predictive Analytics
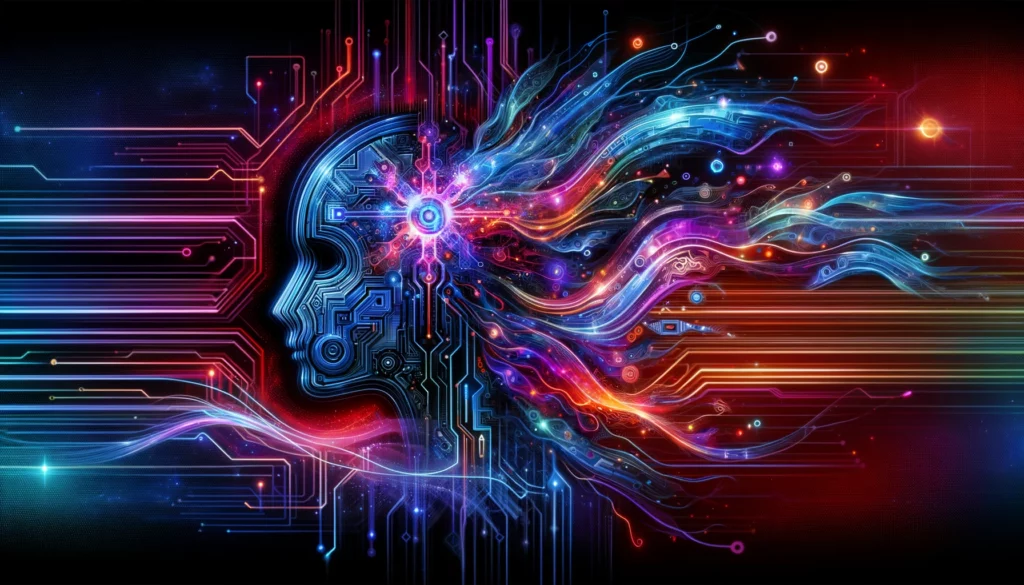
Unlike most modern technologies, Artificial Intelligence stands out due to its autonomous and adaptive nature, offering the ability to streamline threat-hunting processes. By using AI, it is possible to automate various aspects of threat analysis and investigations, freeing up time for more critical tasks.
How AI Benefits Threat Hunting and Analysis
Traditional threat hunting involves laborious manual processes like log analysis and alert reviews, consuming substantial time and effort. However, AI integration allows for efficient automation, enabling real-time detection and the ability to trigger search processes or identify hidden threats promptly. AI systems autonomously review logs, identify patterns in attackers’ tactics, and generate alerts, allowing human analysts to focus on in-depth investigations.
There are numerous advantages to automating threat hunting with AI, providing improved productivity, reduced workload, and enhanced cybersecurity for organizations.
- Rapid Processing Speed. AI boasts unmatched processing speed, swiftly analyzing extensive datasets that would otherwise consume significant time.
- Consistent Accuracy. AI organizes reported threats, ensuring genuine notifications while minimizing the occurrence of false positives that often lead to wasted time.
- Unparalleled Pattern Detection. AI excels in identifying complex patterns, detecting threats that might evade human detection.
- Task Automation. AI reduces the burden of repetitive tasks, enabling professionals to focus on critical responsibilities.
- Constant Adaptation. Machine learning models continuously adapt, staying ahead of evolving threats in the dynamic cybersecurity landscape.
The Role of Artificial Intelligence in Predictive Cybersecurity
Enhancing defenses against evolving cyber threats has become increasingly feasible with the integration of Artificial Intelligence (AI). The role of AI in predictive cybersecurity analytics, primarily driven by machine learning algorithms and predictive analytics, has significantly transformed the landscape of digital security.
Embracing Predictive Cybersecurity Analytics. Predictive cybersecurity analytics, propelled by machine learning algorithms and advanced analytics tools, aims to foresee potential cyber risks before their manifestation. Unlike conventional security measures, predictive analytics identifies and mitigates risks based on trends, anomalies, and historical data.
Using Machine Learning for Threat Identification. AI, particularly machine learning, substantially bolsters threat detection capabilities. By analyzing vast datasets in real-time, machine learning algorithms detect subtle patterns and anomalies that might indicate impending cyber threats. This proactive approach enables organizations to stay ahead of malicious actors and respond promptly to potential threats.
Anomaly Detection and Behavioral Analysis. AI-driven cybersecurity relies on behavioral analysis to understand typical user and system behaviors. Alerts are triggered upon any deviation from established patterns, strengthening defenses against sophisticated cyber threats exploiting vulnerabilities.
Dynamic Risk Assessment. Predictive cybersecurity utilizes AI to perform dynamic risk assessments by evaluating an organization’s risk posture in real time. This assessment factors in user behavior, network traffic, and system vulnerabilities. AI’s adaptability to changing threat landscapes ensures proactive and robust cybersecurity measures.
Incident Response and Threat Mitigation. AI not only aids in threat detection but also significantly impacts incident response and threat mitigation. Automated response systems powered by AI swiftly contain and neutralize threats, minimizing the impact of cybersecurity incidents in a rapidly evolving digital environment.
Continuous Learning and Adaptation. The ongoing learning capabilities of AI benefit predictive cybersecurity by continuously updating machine learning models with fresh data. This adaptability ensures that cybersecurity defenses remain effective against new and unidentified threats.
Integration of Threat Intelligence. Predictive cybersecurity seamlessly integrates threat intelligence feeds with AI systems. This integration enhances predictive capabilities by incorporating real-time data on cyber threats, attack vectors, and vulnerabilities, enabling organizations to proactively counter new risks.
Risk Measurement and Prioritization. AI-driven analytics measure and prioritize risks, extending beyond simple threat detection. Machine learning models assign risk rankings to different activities and potential attacks, allowing cybersecurity teams to focus their attention on critical concerns for efficient resource allocation.
User Behavior Analytics. AI in predictive cybersecurity provides sophisticated analytics on user behavior by establishing standard baselines for user activities. AI models quickly identify variations that may indicate malicious activity or unauthorized access, bolstering cybersecurity defenses and aiding in insider threat detection.
Cybersecurity Flexibility and Reliability. AI-driven predictive analytics play a pivotal role in fortifying cybersecurity resilience. Organizations can adapt to evolving cyber landscapes by preemptively identifying and mitigating potential attacks. Sustaining long-term cybersecurity effectiveness demands this adaptability to keep defenses robust against both current threats and emerging risks.
AI-Powered Threat Detection. Beyond automated detection, AI facilitates proactive threat hunting. Security teams utilize AI techniques to scrutinize historical data, uncovering latent or concealed threats that may not be immediately evident. By preemptively identifying potential threats, this proactive approach adds an additional layer of depth to cybersecurity initiatives.
Compliance Reporting with Regulatory Standards. AI-backed predictive analytics aids businesses in meeting regulatory compliance standards. Through continual monitoring and assessment of cybersecurity threats, organizations generate comprehensive reports validating adherence to industry-specific regulations. This dual function ensures legal compliance while enhancing overall security measures.
Challenges and Ethical Considerations. Despite AI’s potential to revolutionize cybersecurity, there exist obstacles and ethical concerns that warrant attention. As companies embrace these technologies, issues such as algorithmic bias, potential misuse of AI-powered tools, and the necessity for transparent decision-making processes require careful consideration.
Autonomous Decision-Making. AI empowers cybersecurity systems to make autonomous decisions based on learned patterns and established rules. This capability enables swift and autonomous responses to cyber threats, particularly crucial in countering rapid and automated attacks. Balancing autonomy with human oversight ensures responsible decision-making.
Tips for Using AI in Cyber Threat Analysis
While AI-powered threat analysis is effective, several important considerations can optimize its performance.
- Predictive Analytics. Utilize historical and current datasets to feed predictive analytics, improving investigation pace and accuracy.
- Act in a Supporting Role. While AI performs complex analyses, human intervention enhances precision, providing critical thinking where algorithms may lack.
- Reduce Bias and Manipulation. Guard against potential biases and manipulation by training models on secure datasets or using verified open-source resources.
- Generative AI. Consider training AI with synthetic data to simulate potential threats, enhancing preparedness for emerging cyber threats.
By implementing these strategies, professionals can harness the full potential of AI in threat hunting and analysis, fortifying cybersecurity measures in an increasingly complex digital landscape.
AI-Driven Threat Detection Tools
Amazon has transformed the field of threat detection and prevention through its Amazon Web Services (AWS) platform, introducing several AI-powered security solutions.
Among these is AWS GuardDuty, a managed threat detection system that scrutinizes diverse data sources, including AWS CloudTrail logs, VPC Flow Logs, and DNS logs. Its primary objective is to flag any abnormal activity that might signify a security breach. This includes the detection of unusual surges in API calls, irregular network traffic patterns, and unauthorized attempts to access sensitive data. AWS Inspector is another crucial AI-powered service provided by AWS, functioning as a continuous monitoring solution. It aids in pinpointing security vulnerabilities existing within an organization’s AWS infrastructure. Additionally, AWS Macie stands as a groundbreaking service, operating as a fully managed data security solution employing machine learning capabilities. Macie’s functionality involves the discovery, classification, and protection of sensitive data within an AWS environment. Leveraging comprehensive data analysis, Macie identifies crucial information such as personally identifiable information (PII), financial data, and intellectual property (IP).
Darktrace’s Cyber AI Platform The Platform developed by Darktrace employs advanced analytics and machine learning algorithms to identify irregularities in network behavior. Operating in real-time, this platform can uncover concealed vulnerabilities, zero-day exploits, and sophisticated cyber attacks. Utilizing AI-driven analytics, it offers comprehensive visibility into potential threats, enabling proactive measures to address and mitigate these risks before they escalate.
Using cloud-based analytics, platform provides continuous real-time threat monitoring. Beyond recognizing established threats, it employs advanced analytics to forecast potential attack paths. Notably, it excels in monitoring the activities of devices that may be compromised within a network.
System integrates machine learning and extensive data analytics to offer a comprehensive overview of a network’s security status. By examining diverse data sources across an organization’s network, this platform excels in detecting intricate attack patterns and uncovering concealed threats.
By utilizing behavior-based analytics, Cybereason’s platform surpasses traditional signature-based detection methods. Its AI-driven algorithms actively monitor network activities for irregular behavior, issuing early alerts for potential internal or external threats. This capability proves particularly efficient in detecting and neutralizing advanced persistent threats (APTs).
Platform employs machine learning and sophisticated correlation algorithms to deliver an integrated platform for detecting and responding to threats. Capable of analyzing extensive data volumes, it identifies intricate and subtle attack methods, providing analytics both before and after an attack occurs.
Even as cybercriminals leverage advanced technological capabilities, employing AI and machine learning tools to their advantage, cybersecurity measures are evolving to counter the latest threats. From anomaly detection to predictive analytics and risk assessment, a variety of tools offer AI-driven defense for businesses of all scales. By embracing these tools and strategies, cybersecurity professionals can prepare for future threats and adapt to the evolving job market in the field.
Potential Risks of AI in Cybersecurity
The integration of AI into cybersecurity comes with both advantages and potential risks. While it offers significant benefits for cybersecurity professionals, it also presents opportunities for cybercriminals to exploit evolving technologies.
An emerging trend involves cybercriminals leveraging AI and sophisticated analytics to replicate legitimate LLC service websites. Machine learning algorithms scrape extensive data from authentic LLC service sites, enabling them to mimic the visual layout, user flow, and even the specific language used in customer interactions. Using advanced analytics, these fraudulent sites continuously refine their imitation based on user interactions, becoming progressively more convincing over time. This deceptive tactic tricks unsuspecting entrepreneurs into disclosing sensitive personal and financial information, illustrating how AI-powered analytics serve a dual purpose—supporting threat-hunting efforts while empowering cybercriminals to exploit systems meant for protection.
Phishing provides another example of AI’s dual nature. Previously, hackers’ phishing attempts might have been identified by obvious grammatical errors or unnatural language. However, modern cybercriminals leverage Language Models (LLMs) to create highly convincing emails that dupe unsuspecting victims into infecting their devices. AI-generated code makes this feasible without requiring extensive technical knowledge.
Moreover, cybercriminals now utilize AI to rapidly create sophisticated malware with polymorphic attributes, allowing it to evade detection by advanced filters. Despite these concerning developments, cybersecurity experts and white-hat professionals have not remained idle in responding to these emerging threats.