AI’s Self-Adaptive Learning Models
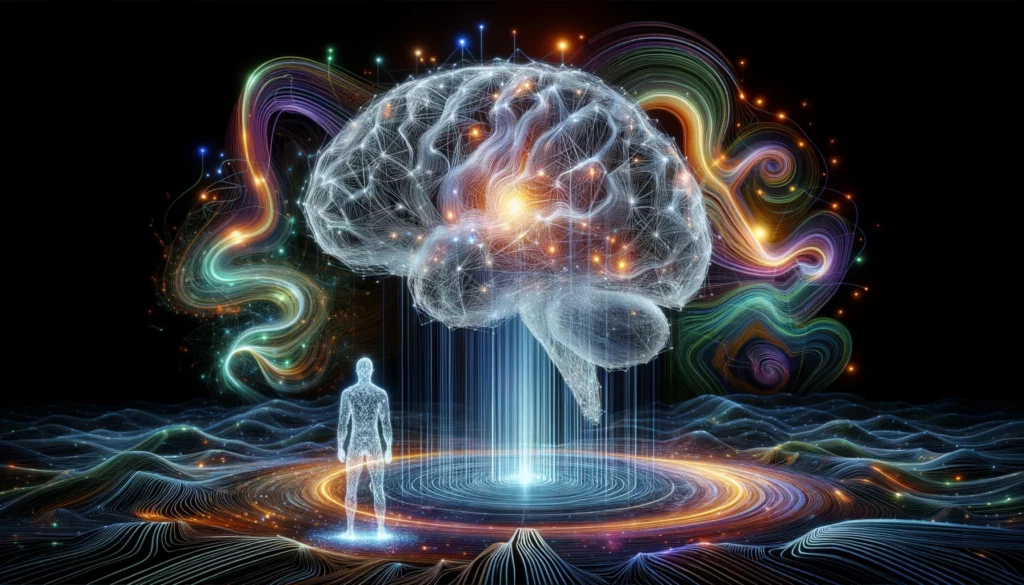
A recent and highly captivating development in the realm of artificial intelligence is the emergence of self-supervised learning. In stark contrast to traditional machine learning models, which heavily depend on labeled data, self-supervised learning empowers AI systems to glean insights from unprocessed data, mirroring the cognitive processes of the human brain. The resemblance between self-supervised learning and the learning mechanisms of the human brain is striking, and it holds the potential to usher in a transformative era for AI applications.
Autonomous Learning Algorithm
Self-supervised learning, a branch of machine learning, empowers AI models to acquire knowledge from extensive datasets without the requirement of human-provided labels. Rather than depending on explicit data annotations, self-supervised models harness data patterns to autonomously generate their own labels, endowing them with remarkable versatility and the capability to effectively manage unstructured datasets.
Key Aspects of Self-Supervised Learning Models
Contrastive Learning. Self-supervised learning often employs contrastive learning, a technique that helps the model differentiate between data points based on their similarities and differences. For example, in image analysis, the model learns to discern whether two different versions of the same image are similar (positive pair) or dissimilar (negative pair). This approach empowers the model to create meaningful data representations.
Language Models. Self-supervised learning has significantly impacted natural language processing (NLP) through models like BERT and GPT. These models are pre-trained on extensive text data, allowing them to grasp the context and semantics of language. Consequently, they have revolutionized tasks such as sentiment analysis, language translation, and question-answering systems.
Applications of Self-Supervised Learning Models
Computer Vision. Self-supervised learning has made substantial strides in computer vision. Models trained using this approach excel in tasks such as image recognition, object detection, and even facial recognition. These models can interpret visual data without the need for human-provided labels, making them adaptable and versatile.
Natural Language Processing. In NLP, self-supervised models like BERT and GPT serve as the foundation for various AI applications. They possess the ability to understand the context, semantics, and sentiment of textual data, enabling applications like chatbots, sentiment analysis tools, and content generation systems.
Recommendation Systems. Self-supervised learning has enhanced recommendation systems across domains such as e-commerce, entertainment, and content platforms. These models can comprehend user behavior patterns and offer product, movie, or content suggestions without relying on explicit user ratings.
Healthcare. Self-supervised learning is making inroads into healthcare by analyzing medical images, predicting disease outcomes, and contributing to drug discovery. These models can make sense of extensive medical data, including images, text, and patient records.
Challenges and Future Prospects
While self-supervised learning has made significant headway, challenges persist, including the need for more efficient model architectures and methods to handle large-scale datasets. Nevertheless, the future of self-supervised learning models appears promising, with anticipated applications spanning autonomous vehicles, personalized medicine, and more.
Self-supervised learning models are reshaping the landscape of AI by enabling machines to learn and comprehend data without human-provided labels. As self-supervised learning models continue to evolve and find new applications, they hold the potential to revolutionize the world and make AI more adaptable and versatile than ever before.