Machine Learning Protection: Safeguarding AI in the Digital Age
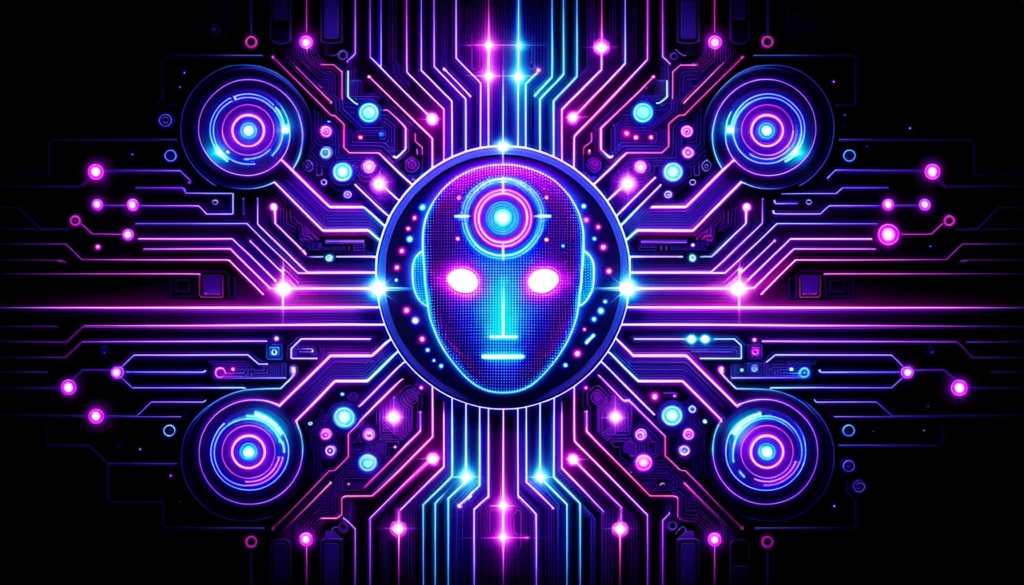
Introduction
In an increasingly digital world, machine learning has become the cornerstone of technological advancement. It powers recommendation systems, autonomous vehicles, fraud detection, and much more. However, with great power comes great responsibility. As the reliance on machine learning grows, so does the need for robust protection mechanisms. In this article, we will explore the critical aspects of machine learning protection, its challenges, and how to ensure the safety and security of this transformative technology.
The Rise of Machine Learning
Understanding the Impact
Machine learning, a subset of artificial intelligence, has revolutionized industries ranging from healthcare to finance. Its ability to analyze vast datasets and make data-driven decisions has led to unprecedented innovations.
The Vulnerabilities
While machine learning has opened new doors, it has also exposed us to vulnerabilities. Attackers can manipulate input data, inject bias, or reverse-engineer models, potentially leading to dire consequences.
The Importance of Protection
Data Security
To protect machine learning models, data security is paramount. Robust encryption and access control measures are necessary to safeguard sensitive training data.
Model Integrity
Ensuring the integrity of machine learning models is crucial. Regular audits and validation checks can detect any unauthorized changes or tampering.
Explainability
Understanding how models arrive at decisions is vital. It not only enhances transparency but also helps in identifying and rectifying biases.
Challenges in Machine Learning Protection
Adversarial Attacks
Adversarial attacks involve manipulating input data to deceive machine learning models. Developing defences against such attacks is an ongoing challenge.
Privacy Concerns
Machine learning often deals with personal data. Striking a balance between innovation and privacy protection is a delicate task.
Scalability
As machine learning applications grow, protecting them at scale becomes increasingly complex. Efficient solutions are required to handle this challenge.
Strategies for Protection
Robust Authentication
Implementing strong authentication mechanisms ensures that only authorized users can access machine learning systems.
Continuous Monitoring
Regularly monitoring machine learning models can help detect anomalies or unusual behaviour, signalling potential threats.
Secure Deployment
Ensuring that machine learning models are deployed in secure environments with proper access controls is essential.
Conclusion
Machine learning protection is not an option; it’s a necessity. As we continue to rely on AI-driven solutions, safeguarding them from threats is our responsibility. By addressing vulnerabilities, understanding the importance of protection, and implementing robust strategies, we can ensure that machine learning continues to drive progress while remaining secure.
FAQs (Frequently Asked Questions)
- What are adversarial attacks in machine learning? Adversarial attacks involve manipulating input data to deceive machine learning models, potentially leading to incorrect decisions.
- Why is model explainability crucial in machine learning protection? Model explainability enhances transparency and helps in identifying and rectifying biases, making machine learning systems more reliable.
- How can I protect sensitive training data in machine learning? Protect sensitive training data through robust encryption and access control measures to ensure data security.
- What challenges does scalability pose in machine learning protection? As machine learning applications grow, protecting them at scale becomes complex, requiring efficient solutions to handle this challenge.
- Why is continuous monitoring important in machine learning protection? Continuous monitoring helps detect anomalies or unusual behaviour in machine learning models, signalling potential threats and ensuring their integrity.